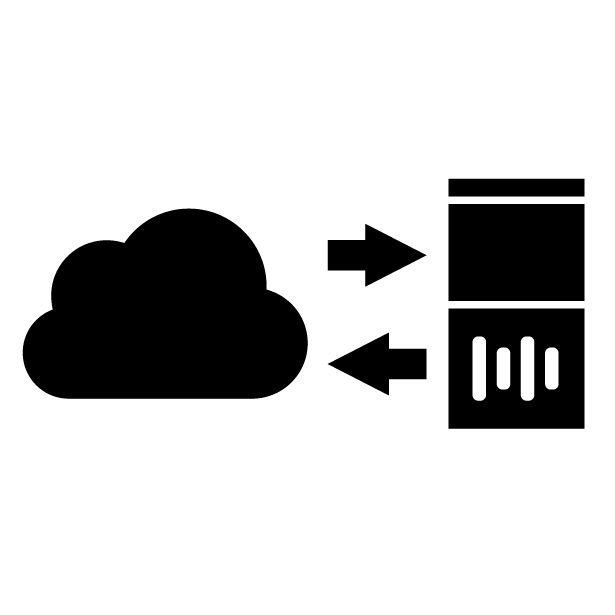
Intelligible Cloud and Edge AI (ICE-AI)
ICE-AI addresses how AI systems can support human trust and ethically-sensitive design. This is done by exploring the development of robust user behaviours and perception of AI in the cloud and AI at the edge.
As AI technology becomes more pervasive, so too do issues around human trust in AI. Without ethically-sensitive design, AI can cause unintended biases. These can affect outcomes and erode human trust. This project considers social and conceptual understandings with user experience of algorithmic systems in the cloud and at the edge.
ICE-AI utilises interviews and observations conducted with users, as well as expert interviews, observations and explorations of people and culture. Lab and user studies using identified cultural problems are also explored. The team aim to develop and evaluate at least one prototype interface.
Project outputs
A study relating to the ICE-AI project was published by authors Auste Simkute, Ewa Luger, Bronwyn Jones, Michael Evans, and Rhianne Jones. The paper, titled “Explainability for experts: A design framework for making algorithms supporting expert decisions more explainable”, maps the decision-making strategies that will be potentially used as explainability design guidelines.
The study argues that the existing explainability approaches lack usability, and are mostly not effective when applied in a decision-making context. In some cases this can result in automation bias and loss of human expertise. For this reason, the authors propose a novel framework with the aim of tailoring to support naturalistic decision-making strategies employed by domain experts and novices.
Read the paper here.
The ICE-AI poster displayed at the PETRAS Academic Conference | Networking Research Showcase on 16 June 2022: