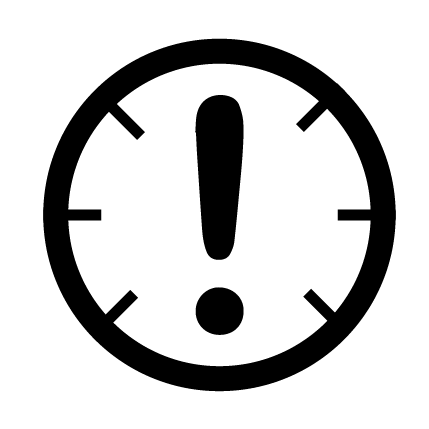
Impact of Cyber Risk at the Edge: Cyber Risk Analytics and Artificial Intelligence (CRatE)
The CRatE project investigated the role of artificial intelligence in predictive cyber risk analytics, with the specific focus on conceptualising the design of an automatic and self-adapting system for anomaly detection. The aim was to mature the quantification of cyber risks in Internet-of-Things systems into a higher Technology Readiness Level (TRL), and the objective was to exhibit how quantitative risk analytics would work in practice.
One of the main obstacles for performing predictive cyber risk analytics on Internet-of-Things systems is the low-memory/low-computational power in such devices. The CRatE project conducted a review of algorithms that could execute artificial intelligence on low memory devices [1]. Second obstacle is the lack of appropriate methodologies for predicting the cyber-attack threat event frequencies and the associated cyber risk loss magnitude. The CRatE project designed a set of mathematical formulas that can be applied for constructing a dynamic and self-adapting system, supported with artificial intelligence, machine learning and real-time intelligence for predictive cyber risk analytics [2]. This work presents one of the main achievements of the CRatE project, because it enables practitioners to use artificial intelligence and machine learning algorithms and statistical approaches for quantifying cyber risk with dynamic risk analytics at the edge. The new solutions derived from integrating a combination of algorithms from the computer science and engineering science domains, in combination with applied mathematics and statistics, adapted to predict the cyber risk from connected IoT devices.
The CRatE project enables the application of machine learning and deep learning algorithms to create new technological capability to predict the cyber risk mean, variance and Value at Risk. The CRatE approach for risk assessment with artificial intelligence algorithms can also be used by practitioners for the prediction of the risk exposure from individual device range i.e., the prediction of the expected shortfall probability, shortfall of expected present value of the loss, conditional tail expectation (in 90%, 95%, and 99%) and the Value at Risk (again in 90%, 95%, and 99%).
The ability to assess cyber risk from Internet-of-Things systems is very important for the adoption of these new low cost/low memory technologies, but it depends on timey probabilistic data. The CRatE project developed a new cognitive cyber physical system capability [3], detailing the process of how new data is captured, stored, processed, analysed, and used in near real-time, with low latency. Building upon this work, the CRatE project advanced this cyber physical approach with production and supply chain concepts from Industry 4.0 [4] enhanced with artificial intelligence and machine learning in dynamic cyber risk analytics at the edge [5]. The cyber risk at the edge is discussed further in the context of dynamic real-time risk analytics of uncontrollable states in complex internet of things systems [6]. This discussion presented a new goal-oriented dependency modelling that can be used by practitioners to assess uncontrollable risk states in complex IoT systems and can also be used for a quantitative self-assessment of IoT cyber risk posture. While the quantification of cyber risks from the Internet-of-Things is further analysed with a new epistemological equation for analysing uncontrollable states in complex systems [7]. The epistemological equation can be used by practitioners in their analysis of the present (the current) and a new target state for IoT systems. The epistemological equation enables practitioners to design a transformation roadmap, describing how IoT systems can achieve the target state with the new epistemological analysis model. The CRatE project also contributed to the design of digital twins, augmented with artificial intelligence and the Internet-of-Things cyber-physical systems – in Industry 4.0. [8].
CRatE in Covid-19 management
Since the research was conducted during the Covid-19 pandemic, the CRatE project engaged in finding new technological and algorithmic solutions for managing Covid-19 and future pandemics i.e., Disease X. The CRatE project explored various practical applications of artificial intelligence for preparing and managing future pandemics. One specific example is the use of artificial intelligence in Internet-of-Things based tracking systems – operating as Social Machines [9]. Building upon the mathematical formulas for quantification of risk, practitioners can apply the same approach to measuring in terms of 90%, 95%, and 99% and categorising different scenarios. This presents the first step in developing the training data for a new algorithm that can process vast amounts of data at speed. Considering that a Disease X event could spread fast, similarly to the Covid-19 pandemics, obtaining reliable data at speed is crucial for the proposed approach to be successful. The lack of timely data problem can be resolved by constructing a new approach for calculating the distribution with real field measurements of Covid-19 speed percentiles, based on Internet-of-Things based tracking systems – operating as Social Machines.
In preparation of such approach being developed in the future, the CRatE project applied statistical analysis (with R programming) to conduct data mining and analysis of Covid-19 mortality, immunity, and vaccine development [10]. This research presents a snapshot in time from the first wave of the Covid-19 pandemic, which can be beneficial for constructing training scenarios for the proposed algorithm. The CRatE project reviewed the ethics of shared Covid-19 risks and constructed a new epistemological framework for ethical health technology assessment of risk in vaccine supply chain infrastructures [11]. Building upon this work, the CRatE project conducted epistemological and bibliometric analysis of ethics and shared responsibility aimed at guiding the decision makers in creating a new health policy for Internet-of-Things systems in healthcare [12]. The CRatE project also created a new methodology for integrating artificial intelligence in healthcare systems, to guide healthcare practitioners in learning from COVID-19 to prepare for Disease X [13]. The CRatE project also contributed to the discussion on alternative mental health therapies in prolonged lockdowns, building upon digital narratives from Covid-19 [14].
CRatE in technical magazines
The CRatE project has attracted a lot of attention from the scientific community by communicating and disseminating results and findings through news articles in technical magazines, such as IEEE Future Directions and IEEE Internet of Things. For example, the CRatE project participated in the discussion on assessing the cyber risk from using IoT devices in managing Covid-19 [15]. This discussion was published in a research article, detailing the construction of a new approach for integrating AI in the risk analytics of medical IoT solutions, creating a stronger resilience of systems through cognition in their physical and digital dimensions. This approach created a new understanding on how AI can enable medical IoT systems to recover in response to adverse events. Enabling a design of a similar model for future pandemic management, assisting medical IoT systems to continuously adapt, requires employing AI techniques to understand and mitigate the vulnerabilities of adverse events.
The CRatE project continued building upon this topic and has produced an article on a new cloud IT operating model for pandemic management [16]. The model was partially based on earlier work on cyber risk from IoT devices and networks [17] and cloud technologies [18].
References:
Journal articles on cyber risks in Internet-of-Things systems
[1] Radanliev, Petar., and de Roure, David, “Review of Algorithms for Artificial Intelligence on Low Memory Devices,” IEEE Access, vol. 9, pp. 109986–109993, 2021, doi: 10.1109/ACCESS.2021.3101579, URL: https://ieeexplore.ieee.org/document/9502714.
[2] Radanliev, Petar., De Roure, David., Page, Kevin., Van Kleek, Max., Santos, Omar., Maddox, La’Treall., Burnap, Peter., … Maple, Carsten, “Design of a dynamic and self-adapting system, supported with artificial intelligence, machine learning and real-time intelligence for predictive cyber risk analytics in extreme environments – cyber risk in the colonisation of Mars,” Saf. Extrem. Environ., vol. 2, no. 3, pp. 219–230, Feb. 2021, doi: 10.1007/s42797-021-00025-1, URL: http://link.springer.com/10.1007/s42797-021-00025-1.
[3] Radanliev, Petar., De Roure, David., Van Kleek, Max., Santos, Omar., and Ani, Uchenna, “Artificial intelligence in cyber physical systems,” AI Soc., vol. 1, pp. 1–14, Aug. 2020, doi: 10.1007/s00146-020-01049-0, URL: https://doi.org/10.1007/s00146-020-01049-0.
Dr Petar Radanliev has written a blog post about this publication here.
[4] Radanliev, Petar., De Roure, David., Nicolescu, Razvan., Huth, Michael., and Santos, Omar, “Artificial Intelligence and the Internet of Things in Industry 4.0,” CCF Trans. Pervasive Comput. Interact., pp. 1–10, Mar. 2021, doi: 10.1007/s42486-021-00057-3, URL: http://link.springer.com/10.1007/s42486-021-00057-3.
[5] Radanliev, Petar., De Roure, David., Walton, Rob., Van Kleek, Max., Montalvo, Rafael Mantilla R.M., Maddox, La’Treall L.T., Santos, Omar., … Anthi, Eirini, “Artificial intelligence and machine learning in dynamic cyber risk analytics at the edge,” SN Appl. Sci., vol. 2, no. 11, pp. 1–8, Nov. 2020, doi: 10.1007/s42452-020-03559-4, URL: https://doi.org/10.1007/s42452-020-03559-4.
[6] Radanliev, Petar., De Roure, David., Van Kleek, Max., Ani, Uchenna., Burnap, Pete., Anthi, Eirini., Nurse, Jason R.C., … Maddox, La’Treall T., “Dynamic real-time risk analytics of uncontrollable states in complex internet of things systems: cyber risk at the edge,” Environ. Syst. Decis., vol. 41, no. 2, pp. 236–247, Nov. 2020, doi: 10.1007/s10669-020-09792-x, URL: https://doi.org/10.1007/s10669-020-09792-x.
[7] Radanliev, Petar., De Roure, David., Burnap, Pete., and Santos, Omar, “Epistemological Equation for Analysing Uncontrollable States in Complex Systems: Quantifying Cyber Risks from the Internet of Things,” Rev. Socionetwork Strateg. 2021, pp. 1–31, Jul. 2021, doi: 10.1007/S12626-021-00086-5, URL: https://link.springer.com/article/10.1007/s12626-021-00086-5.
[8] Radanliev, Petar., De Roure, David., Nicolescu, Razvan., Huth, Michael., and Santos, Omar, “Digital twins: artificial intelligence and the IoT cyber-physical systems in Industry 4.0,” Int. J. Intell. Robot. Appl., pp. 1–15, May 2021, doi: 10.1007/s41315-021-00180-5, URL: https://link.springer.com/10.1007/s41315-021-00180-5.
Journal articles on Covid-19
[9] Radanliev, Petar., De Roure, David., Walton, Rob., Van Kleek, Max., Montalvo, Rafael Mantilla., Santos, Omar., Maddox, La’Treall T., and Cannady, Stacy, “COVID-19 what have we learned? The rise of social machines and connected devices in pandemic management following the concepts of predictive, preventive and personalized medicine,” EPMA Journal, vol. 11, no. 3. Springer, pp. 311–332, 01-Sep2020, doi: 10.1007/s13167-020-00218-x, URL: https://doi.org/10.1007/s13167-020-00218-x.
Dr Petar Radanliev has written a blog post about this publication here.
[10] Radanliev, Petar., De Roure, David., and Walton, Rob, “Data mining and analysis of scientific research data records on Covid-19 mortality, immunity, and vaccine development – In the first wave of the Covid-19 pandemic,” Diabetes Metab. Syndr. Clin. Res. Rev., vol. 14, no. 5, pp. 1121–1132, Sep. 2020, doi: 0.1016/j.dsx.2020.06.063, URL: https://doi.org/10.1016/j.dsx.2020.06.063.
[11] Radanliev, Petar., De Roure, David., Ani, Uchenna., and Carvalho, Graca, “The ethics of shared Covid-19 risks: an epistemological framework for ethical health technology assessment of risk in vaccine supply chain infrastructures,” Health Technol. (Berl)., pp. 1–9, Jun. 2021, doi: 10.1007/s12553-021-00565-3, URL: https://link.springer.com/article/10.1007/s12553-021-00565-3.
[12] Radanliev, Petar., and De Roure, David, “Epistemological and Bibliometric Analysis of Ethics and Shared Responsibility—Health Policy and IoT Systems,” Sustainability, vol. 13, no. 15, 2021, doi: 10.3390/su13158355, URL: https://www.mdpi.com/2071-1050/13/15/8355.
[13] Radanliev, Petar., De Roure, David., Maple, Carsten., and Ani, Uchenna, “Methodology for integrating artificial intelligence in healthcare systems: learning from COVID-19 to prepare for Disease X,” AI Ethics 2021, vol. 1, pp. 1–8, Oct. 2021, doi: 10.1007/S43681-021-00111-X, URL: https://link.springer.com/article/10.1007/s43681-021-00111-x.
[14] Radanliev, Petar., and De Roure, David, “Alternative mental health therapies in prolonged lockdowns: narratives from Covid-19,” Heal. Technol. 2021, pp. 1–7, Aug. 2021, doi: 10.1007/S12553-021-00581-3, URL: https://link.springer.com/article/10.1007/s12553-021-00581-3.
News articles on technical magazines
[15] Radanliev, Petar., De Roure, David., and Van Kleek, Max, “Cyber-risks from Using IoT Devices for Managing COVID-19,” IEEE IoT Newsletter, 2021, URL: https://iot.ieee.org/newsletter/january-2021/cyber-risks-from-using-iot-devices-formanaging-covid-19.
[16] Radanliev, Petar., Roure, Dave De., and De Roure, David, “Covid-19 and cloud technologies: All-Cloud IT Operating Model for pandemic management – Part 2,” IEEE Future Directions, Jan-2021, URL: https://cmte.ieee.org/futuredirections/techpolicy-ethics/march-2021/covid19-and-cloud-technologies-part2/.
[17] Radanliev, Petar, “Cyber Risk from IoT Devices and Networks,” IEEE Internet of Things Newsletter, 2020, URL: https://iot.ieee.org/newsletter/july-2020/cyber-riskfrom-iot-devices-and-networks.
[18] Radanliev, Petar., and De Roure, David, “Cloud technologies: All-Cloud IT Operating Model,” IEEE Future Directions, 2020, URL: https://cmte.ieee.org/futuredirections/tech-policy-ethics/september-2020/cloudtechnologies-all-cloud-it-operating-model/.